I recently had a chance to review Meltdown: Why Our Systems Fail and What We Can Do About It, which takes a critical look at several examples of catastrophic failure in many differing areas and applies Perrow’s theories of Normal Accidents in order to address these systemic problems we face regularly. A lot of the background in human factors I’m familiar with takes a different tact from Perrow’s, but I was hoping for surprising new insights into areas I hadn’t yet considered when examining safety. Sadly, I can’t say that to be the case. Meltdown reinforces and distorts theories on safety that have long been shown to have flawed approaches towards investigating how our systems fail and how we can learn from these failures.
A quick primer on Perrow’s theory of “Normal Accidents”
Meltdown attempts to characterize how our systems fail with example after example of mostly modern day scenarios, be it in tech, transportation, or medicine, to illustrate how they’re all connected in failure. The school of thought that makes up the vast bulk of this work comes from Charles Perrow who is notable for his investigative work into the catastrophe at Three Mile Island as well as emeritus professor at Yale and the author of several works in safety investigation, including Normal Accidents. His theories, simplified for brevity, are summarized as follows:
A system can be described on two axes: how interactive the components of a system are (linear vs. complex) and how coupled distinct elements are (loosely or tightly).
Linear systems are straight forward whereas complex systems have many interacting parts that have feedback loops.
Loosely coupled systems have “natural” barriers while tightly coupled systems do not. A tightly coupled system can therefore be characterized by quick and often strong changes between related components.
Below is a chart indicating examples of systems that demonstrate such:
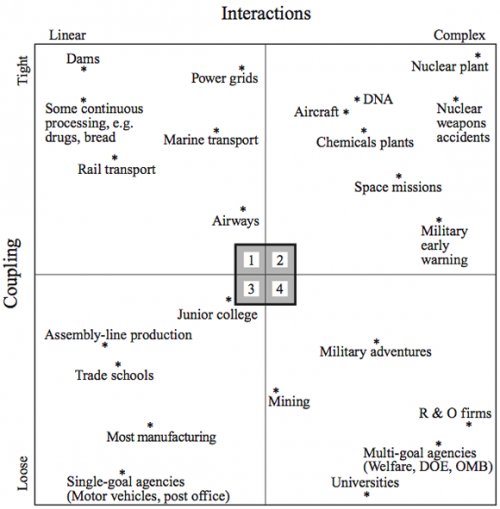
So what solutions do we have for problems within these systems? Perrow says if a system is too tightly coupled, the control structure must be centralized to compensate for it. If it is too complex, it must be decentralized so that their component parts are better understood in a smaller scope. The greatest risk lies in systems that are both tightly coupled and complex (say, a nuclear plant). In those cases, he argues you can’t both centralize and decentralize the system, so accidents will be “normal”. In this case, “normal” means that though they can happen infrequently they are expected as they can not be controlled no matter how you organize.
Many other theories, including those I subscribe to, push back on Perrow’s. In particular is a document from SINTEF comparing several approaches to systems safety, Organisational Accidents and Resilient Organisations: Six Perspectives. Some of the weaknesses in Perrow’s thinking from the paper are:
- The notions of “interactive complexity” and “tight coupling” are so vague that it is difficult or impossible to subject the theory to empirical tests.
- It is difficult to derive a simple and effective prescription for assessing or monitoring major accident risk from Normal Accident theory, because it is difficult to measure or monitor such attributes as “interactive complexity” or “decentralisation”.
- Analysis of recent major accidents suggests that most accidents result from other problems than a mismatch between complexity/coupling and degree of centralisation (Hopkins, 1999).
- Some critics find the suggestion that some technologies should be discarded too pessimistic, too fatalistic, or politically unacceptable.
- The assertion that an organisation cannot be centralised and decentralised at the same time sounds like a tautology. However, this assertion has been challenged by researchers that study so-called High Reliability Organisations (Weick, 1987).
With that in mind, I disagree with the fundamental basis for Meltdown.
Perrow’s theories in Meltdown
Returning to the book, there are several sections where the authors (András Tilcsik and Chris Clearfield) attempt to clarify how Perrow’s theories interact with systems all around us and our negligence to either notice the signs of failure creeping up or design systems that are too complex are our downfall. Repeatedly throughout the book they will describe an event, indicate its unfortunate outcome in great detail to emphasize just how bad things were, and they mention “complexity!” as if that is the one answer to the problem. Some questions I’ve had in mind while reading this:
- How complex is “too” complex, and how do you go about measuring such?
- Where are the systems we’re looking at that are complex but aren’t failing us in these catastrophic ways, and the linear systems on the other end that do fail?
- At what point do we agree, and how do we come to an agreement, that a system we’ve built is too complex?
- Is it really complexity, or the understanding of a system, that is the problem?
- Is complexity relative? If we learn a system and understand it, is it still too complex for its own good? (Short answer to this: people are part of the system, so the system has changed!)
In all, the point is supposed after the fact, which is an indication of hindsight thinking. They find situations where a major failure has occurred and diagnose it as complex afterwards, cherry picking situations that fit their theory. There is never a given account of the decisions made along the way or why individuals build structures that necessitate the complexity as described. It’s also much easier to characterize a system as complex from the outside looking in, which heightens support for things being “too complex to understand”.
Blameful Language
Early on in the book, Tilcsik and Clearfield go into some explanation of their own into Perrow’s theory, describing coupling and complexity, as a setup for the rest of the book. In particular, one section left me particularly sour on their assumptions:
“Most disasters are preventable, and their immediate causes aren’t complexity and tight coupling but avoidable mistakes – management failures, ignored warning signs, miscommunications, poor training, and reckless risk-taking.” (pg. 27)
There’s so much wrong in one sentence I had to take a second and third read over it to make sure I hadn’t missed something. In particular:
- “Preventable” This misses the entire point of a learning exercise. That’s a judgement call made with hindsight bias in full effect. The embarrassing part is they mention hindsight bias and yet fall so completely for it themselves.
- “Their immediate causes aren’t complexity and tight coupling but avoidable mistakes” The entire premise of the book (complexity/tight coupling cause accidents) is undermined by stating most disasters aren’t due to those two factors.
- “Reckless” Another judgement call after the fact. Skipping your testing suite can be considered “reckless”. Were you in a major outage where returning to a stable state warranted immediate action? There’s context to be had that language like this hides.
- “Poor training”. Even experts get things wrong. Failure happens to all of us. “Training” won’t prevent all failures (failures are always happening!).
- “Ignored warning signs” We have to filter out countless tiny warnings a day, indications of what is “normal” and “abnormal”. Warning signs are very obvious after an outage because we think of things in terms of linear causality, when in fact we have to deal with near infinite possibilities when making a decision. Only in retrospect do we see which are valuable and which are ok to ignore.
Accident vs. Malice
Scattered throughout the book are anecdotes from all walks of life describing failure and the systems involved. Many of them are frequently discussed stories from the field of safety, such as Three Mile Island nuclear accident and the Knight Capital software glitch that cost them $450 million in half an hour. These are real world scenarios that are worthy of investigation. Unfortunately, Tilcsik and Clearfield cite several examples that aren’t cases of systems failing in unexpected ways but acts of theft, malice, and cover ups to hide wrongdoing as a means of bolstering their argument that complex systems are evil.
In particular, they walk through the intentional ignorance of the water crisis in Flint Michigan, Enron’s financial juggling, a reporter for the New York Times falsifying stories, and Volkswagen’s cheating with emissions during testing. These are all poor examples to use in comparison to safety management in complex systems. The link between an event like Three Mile Island and the above malfeasance is that all involve complex, highly interconnected systems. Therefore…complex systems must be at fault. This is a reach at best, but much more likely poor scientific research to argue a point.
Human Factors research emphasizes people as a key component to keeping our systems up and running every day despite countless tiny failures that pop up under constantly changing situations. In accident scenarios, people do not seek to undermine or invalidate safety protocols for profit. We examine the safety of our systems because despite people’s best intent to be safe under different pressures, we tend to choose the path of least resistance that will accomplish our goals (see The ETTO Principle). By conflating this moral bankruptcy (intentionally deceiving) with best efforts to achieve goals (misunderstanding), we revert to retributive justice thinking. Everyone must be trying to undermine the rules and therefore the rules must be held at all costs. Restorative justice (aka “Just Culture”) instead places an emphasis on understanding how we can help people better understand their complex systems, rather than blame them for when things go wrong. These examples are at odds with learning culture. The book in illustrating these only encourages people to hide mistakes and prevent real learning from happening.
Failing to Propose Meaningful Solutions
Understanding complex systems is a hard problem, requiring deep thoughtfulness and inspection. In that premise, I do agree with Tilcsik and Clearfield. Their fundamental misunderstanding of systems, though, means their conclusions are wrong, and so the proposed solutions given in the book are not entirely effective. Hindsight bias is strong here, looking at how problems were “avoidable” and warning signs were “clear” and “obvious”. This thinking runs counter to a learning culture as well, shaming folks for not being able to predict the future. One example relates to the Fukushima nuclear accident (pg 100) where they cite “it was preventable” as the walls were too short (only 10 ft tall, unable to withstand 13 ft waves). There is no discussion here on the decision making about why 10 ft tall walls were considered enough and the cost too great to buildthem higher. Why not make them 20 ft? 30 ft? 100 ft? Language like this is prevalent in the book, cutting the legs out of the important parts of understanding our systems.
To their credit, there are meaningful approaches to learning and growing in organizations that they do mention, which I commend them on. In particular, they devote an entire chapter to diversity as ones means of improvement for a complex system. It would be unfair of me to diminish this, something so crucial for us both as an ethical obligation as well as a means of improving how we go about our work. This can (and has been) the source of many scholarly articles and books, rightfully so. Addressing it in a book otherwise devoted to understanding complex systems is ambitious. I would love for more on this, but a book can only be so long.
Diversity as written about in this book, though, is tied in with dissent as a means of challenging ideas and topics. Homogeneous teams tend to agree without critical thinking, which is argued to be potentially influencing disasters. This is where the topic gets muddled. The discomfort we feel with heterogeneous teams (as in, we all don’t look alike and our inclination is to agree with people who like like ourselves) means we question more, but they don’t directly show that dissent and asking tougher questions immediately gives more transparency to complex systems. It is, again, a shallow answer. Challenge more ideas, something something something, and now our systems are either not so complex or are more transparent.
Anomalizing is a term used in reference to the approach in learning from the information our systems are showing us as a means of managing complexity. It’s a cyclic routine consisting of 5 steps: Gather, Fix, Find Root Causes, Share, and Audit. This seems like a process that should be much more highlighted in the book, but they spend 6 pages on it, half of which is a story on a company applying some of the theory. I’m also an opponent of root cause (or causes) as an explanation for failures in our system, believing them to be shallow explanations when we don’t wish to understand the interrelated components of our system. As the authors believe tightly coupled and highly complex systems are so dangerous to work in, I would hope they would have come to a similar conclusion. I’m also very confused as to how you can gather information, fix them, then find the “root cause”, followed by sharing and an audit. The waterfall model for diagnosing and understanding a system falls apart very quickly.
Conclusion
We need more research in the field of complex systems. The authors, with seemingly the best of intentions, fail to include much of the modern day thinking surrounding safety, instead citing parts of Perrow’s work and then hand waving over solutions on how to address. Counterfactual thinking and hindsight bias with good intentions confuses and undermines the critical thinking we need in the field of safety.
People are what allow systems to adapt to every changing stimuli that would otherwise sideline the confluence of failures within them. The study of safety must therefore center on people if we’re to understand the systems we’re a part of.
(header image from: https://www.flickr.com/photos/notjake/5399563391)